COO Magazine Q4 2024
AI Friend and Foe: AI Technologies Represent a Tremendous Opportunity and Threat in Managing Non-Financial Risks
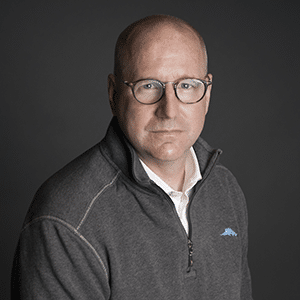
Chris Rigg
Armstrong Wolfe Alliance Partner
Global Economics Group
The Phantom Conference
Sarah Chen sighed as she settled into her chair, preparing for yet another video conference. These calls had become routine for her as a senior financial analyst at Arup’s Hong Kong office, but this one felt different from the start.
The urgent message from the UK-based CFO had been marked “confidential.” As faces flickered onto the screen, Sarah felt a twinge of unease. There was James, the CFO, looking as stern as ever. And was that Melissa from accounting? The London office backdrop looked spot-on.
“Sarah, we need your help with a delicate matter,” James began, his voice carrying that familiar hint of Manchester in his accent. “We’re finalizing a major acquisition, but it needs to stay off the books for now. I need you to make some transfers. Quickly and quietly.”
Sarah’s fingers hovered over her keyboard. Something felt off, but she couldn’t quite place it. The faces, the voices – everything seemed real. And yet…
“Of course, sir,” she heard herself say. “I’ll take care of it right away.”
Over the next few days, Sarah made the transfers as instructed—fifteen in total—to five different Hong Kong accounts. With each one, a nagging doubt grew in the back of her mind.
Finally, unable to shake her suspicions, Sarah called the London headquarters. Her blood ran cold as the truth unraveled. There had been no conference call. No secret acquisition. The James she’d seen was a digital phantom, a deepfake crafted by skilled criminals.
As the reality of the $25 million fraud sank in, Sarah felt a mix of horror and fascination. How had they done it? The voices and mannerisms were all perfect replicas. She’d been fooled by ghosts in the machine, digital doppelgangers who had danced across her screen.
In the days that followed, as police combed through logs and Arup’s IT team fortified their defenses, Sarah couldn’t shake one chilling thought: in a world where seeing was no longer believing, who could you trust?
The Growing Landscape for Non-Financial Risks
When news of the Arup breach reached the world, it was clear that we had entered a new era of risk driven by artificial intelligence technology’s power and availability. Global banks face an increasingly complex array of non-financial risks that can significantly impact their operations, reputation, and bottom line. The international banking industry has incurred over $460 B in losses attributed to non-financial risks, with $19 B occurring in 2022. These risks include operational risks, compliance risks, cybersecurity threats, reputational risks, and more. Non-financial risks have become equally critical in recent years due to several factors:
• Increasing regulatory scrutiny and compliance requirements
• The rapid pace of technological change and digital transformation
• Growing cybersecurity threats and data privacy concerns
• Heightened reputational risks in the age of social media
• The need for operational resilience in the face of disruptions
As a result, banks must take a more holistic and proactive approach to risk management that encompasses both financial and non-financial risks. AI and ML technologies are proving to be invaluable tools in this endeavor. These advanced tools offer robust risk identification, assessment, monitoring, and mitigation capabilities.
Emerging AI Technologies Provide Critical Risk Management Capabilities
Banks can leverage key emerging AI technologies to better manage non-financial risks, including digital twins, machine learning, natural language processing, and advanced analytics. Digital twins stand out as a powerful tool, allowing banks to create virtual replicas of their operations and risk landscapes for real-time monitoring and scenario analysis. Machine learning algorithms can detect anomalies and patterns in vast datasets, enhancing fraud detection and operational risk management. Natural Language Processing (NLP) enables banks to analyze unstructured data from various sources, improving compliance monitoring and reputational risk assessment. Deep learning models can be applied to complex risk modeling and predictive analytics.
Real-time analytics platforms allow for continuous monitoring of transactions and activities, enabling immediate risk detection and response. Generative AI and Large Language Models (LLMs) can process and analyze vast amounts of textual data to identify potential risks and generate insights. Additionally, explainable AI (XAI) technologies are crucial for ensuring transparency in AI-driven risk decisions, essential for regulatory compliance. By integrating these advanced AI technologies, banks can create a more comprehensive, proactive, and adaptive approach to managing non-financial risks in an increasingly complex operating environment.
Critical Applications of AI in Non-Financial Risk Management
AI-powered systems are being deployed to analyze vast amounts of structured and unstructured data from various sources, enabling more effective identification and assessment of operational, reputational, and compliance risks. For instance, machine learning algorithms detect anomalies in transaction patterns that may indicate fraud or money laundering, significantly improving the accuracy and speed of fraud detection. Natural Language Processing (NLP) techniques monitor social media and news sources for potential reputational threats, allowing banks to respond proactively to emerging issues. In regulatory compliance, AI systems automate the review of complex regulatory documents, ensuring banks stay up-to-date with changing requirements and identify potential compliance gaps.
Additionally, AI-driven predictive analytics enhance scenario analysis and stress testing capabilities, helping banks better prepare for potential risks. By automating routine tasks and providing deeper insights, AI is improving the efficiency of risk management processes and enabling risk teams to focus on more strategic, high-value activities. This shift towards AI-enabled risk management is helping banks build more robust, proactive, and comprehensive approaches to managing non-financial risks in an increasingly complex operating environment.
1. Semantic Analysis to Enhance Risk Identification and Assessment
Semantic analysis can significantly enhance a bank’s ability to identify and classify emerging risks by providing deeper insights into unstructured data from various sources. By leveraging natural language processing and machine learning techniques, semantic analysis can go beyond simple keyword matching to understand textual information’s context, meaning, and relationships. This allows banks to detect subtle linguistic cues and patterns indicating emerging risks, even when explicit terms are not used. Semantic analysis can process vast amounts of data from internal documents, regulatory filings, news articles, social media, and other external sources, identifying connections between concepts that might not be immediately apparent to human analysts. It can also help categorize risks more accurately by understanding the nuanced differences between risk types and mapping them to existing risk taxonomies.
Additionally, semantic analysis can track the evolution of risk-related discussions over time, enabling banks to identify trending topics and weak signals that could develop into significant threats. Semantic analysis systems can become even more adept at recognizing industry-specific risks by incorporating domain-specific knowledge and ontologies. This comprehensive approach allows banks to create a more dynamic, proactive, and accurate risk identification and classification process, ultimately improving their overall risk management capabilities.
AI and ML algorithms can analyze vast amounts of structured and unstructured data from diverse sources to identify potential risks that traditional methods may miss. This includes:
• Natural language processing (NLP) to scan news articles, social media, and regulatory documents for early warning signs of emerging risks
• Pattern recognition to detect anomalies in transaction data, customer behavior, or operational processes that may indicate risks
• Predictive modeling to forecast potential future risks based on historical data and current trends
Leveraging these capabilities allows banks to gain a more comprehensive and nuanced understanding of their risk landscape, allowing for more accurate risk assessments and prioritization of mitigation efforts.
2. Real-Time Risk Monitoring and Alerting
AI technologies can analyze and monitor non-financial risks in real time by implementing a comprehensive, data-driven approach that harnesses the power of machine learning, natural language processing, and predictive analytics. Advanced AI systems can continuously ingest and analyze vast amounts of structured and unstructured data from diverse sources, including internal transaction records, customer interactions, social media feeds, news articles, and regulatory updates. Natural Language Processing techniques enable extracting relevant information from textual data, allowing for the identification of subtle linguistic cues and patterns that may indicate emerging risks. Machine learning algorithms can detect anomalies and unusual patterns in real time, flagging potential operational, reputational, or compliance issues before they escalate.
AI-powered sentiment analysis can monitor public opinion and media coverage, providing early warning of potential reputational risks. Additionally, predictive analytics models can assess the probability of various risk scenarios based on current data and historical trends, enabling proactive risk mitigation. By integrating these AI capabilities with existing risk management systems, banks can create dynamic risk scoring models that continuously update based on real-time data, providing risk managers with instant alerts and a more accurate, current view of the bank’s risk exposure.
This AI-driven approach allows banks to move beyond periodic risk assessments to a more agile, responsive, and comprehensive risk management strategy, ultimately enhancing their ability to identify, assess, and mitigate non-financial risks in real time. This enables banks to:
• Detect and respond to potential issues more quickly
• Identify patterns or trends that may signal emerging risks
• Generate automated alerts when predefined risk thresholds are breached
Real-time monitoring is particularly valuable for managing operational risks, fraud detection, and cybersecurity threats where rapid response is critical.
3. Improved Compliance Management Through Digital Twins
Digital twin technology can significantly enhance their management of compliance obligations by creating a virtual replica of their entire regulatory landscape. This digital twin can integrate real-time data from various sources, including internal systems, regulatory feeds, and external market data, to provide a comprehensive and dynamic view of the bank’s compliance status. By simulating different scenarios and regulatory changes, the digital twin allows banks to proactively assess the impact of new regulations or modifications to existing ones on their operations, processes, and systems. This enables compliance teams to identify potential issues before they arise and develop more effective strategies for maintaining regulatory adherence.
The digital twin can also automate compliance reporting by continuously monitoring transactions and activities against regulatory requirements, flagging potential violations in real time, and generating automated reports for internal and external stakeholders. Furthermore, it can facilitate more efficient and accurate stress testing and scenario planning for regulatory purposes, helping banks demonstrate their resilience and compliance to regulators. By providing a centralized, up-to-date view of the bank’s compliance posture, the digital twin enables better collaboration between different departments and more informed decision-making at all levels of the organization. Ultimately, this technology empowers banks to move from a reactive to a proactive compliance management approach, reducing the risk of regulatory breaches and associated penalties while optimizing resource allocation for compliance activities.
4. Enhanced Cybersecurity and Fraud Detection
AI and ML are potent tools for strengthening banks’ defenses against cyber threats and fraud. Machine learning algorithms can analyze vast amounts of data in real time, identifying patterns and anomalies that may indicate cyber threats or fraudulent activities. These systems can continuously learn and adapt to new attack vectors and fraud schemes, staying ahead of evolving threats. Natural Language Processing (NLP) can analyze unstructured data from various sources, including emails, chat logs, and social media, to detect potential phishing attempts or social engineering attacks. Deep learning models can process complex datasets to identify subtle indicators of fraud that might escape traditional rule-based systems. AI-powered behavioral analytics can establish user and system baseline patterns, flagging deviations that could signal a security breach or fraudulent activity.
Additionally, AI can automate threat intelligence gathering and analysis, enabling faster response times to emerging cyber threats. In fraud detection, AI can monitor real-time transactions, assign risk scores to transactions, and automatically flag suspicious activities for further investigation. Banks can leverage these advanced AI technologies to create a more robust, proactive, and adaptive approach to cybersecurity and fraud prevention, significantly reducing their risk exposure in an increasingly complex digital landscape.
Key applications include:
• Behavioral analytics to detect unusual patterns that may indicate fraud or cyber attacks
• Anomaly detection to identify potential security breaches or unauthorized access attempts
• Predictive modeling to anticipate and prevent potential cyber threats
• Automated threat intelligence gathering and analysis
By leveraging AI, banks can stay ahead of evolving cyber risks and fraud schemes, protecting their assets and customers’ data.
5. Digital Twins for Operational Risk Management
Digital twins can significantly enhance operational resilience by providing organizations with a comprehensive, real-time virtual replica of their operational ecosystem. This technology allows banks to create detailed models of their processes, systems, and infrastructure, enabling them to simulate various scenarios and stress test their operations without impacting real-world activities. By integrating data from multiple sources, digital twins offer a holistic view of the organization’s risk landscape, helping identify vulnerabilities and dependencies that might otherwise go unnoticed. This capability is particularly valuable for anticipating and mitigating the impacts of potential disruptions, such as cyberattacks, system failures, or regulatory changes.
Digital twins enable banks to conduct more sophisticated and realistic scenario planning, moving beyond traditional tabletop exercises to dynamic, data-driven simulations. This allows for more effective contingency planning and helps optimize response strategies. Furthermore, by providing a centralized, up-to-date view of the bank’s operational status, digital twins facilitate better collaboration between different departments and more informed decision-making at all levels of the organization. Ultimately, digital twins empower banks to shift from a reactive to a proactive approach to managing operational risks, enhancing their ability to maintain critical functions and services despite severe disruptions, thereby significantly improving their overall operational resilience.
6. Reputational Risk Management
Natural Language Processing (NLP) and sentiment analysis algorithms can continuously scan and interpret vast amounts of data from social media, news outlets, customer feedback, and other online sources. These AI systems can detect subtle shifts in public sentiment, identify emerging issues or potential crises, and flag negative mentions or discussions about the bank. Machine learning models can be trained to recognize patterns that may indicate reputational threats, allowing for early detection and proactive response. AI-powered predictive analytics can assess the potential impact of various scenarios on the bank’s reputation, enabling more informed decision-making in crisis management. Additionally, AI can automate the process of generating reputation reports and alerts, ensuring that relevant stakeholders are promptly informed of any significant developments. By integrating these AI capabilities with human expertise, banks can create a more robust, proactive, and responsive approach to managing reputational risks, ultimately safeguarding their brand image and maintaining stakeholder trust in an increasingly complex and fast-paced digital landscape.
Conclusion
Artificial intelligence and machine learning offer powerful tools for global banks to enhance their management of non-financial risks. By leveraging these technologies, banks can gain deeper insights into their risk landscape, automate routine risk management tasks, and respond more quickly and effectively to emerging threats. However, successful implementation requires careful planning, investment in data and technology infrastructure, and a commitment to responsible and ethical use of AI.
As the banking industry evolves, institutions that effectively harness AI for comprehensive risk management will be better positioned to navigate an increasingly complex and uncertain business environment. By embracing these advanced technologies, global banks can mitigate risks more effectively and unlock new opportunities for growth, innovation, and competitive advantage in the digital age.
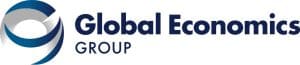